Home Page
The MIT Press Reader
Read more on the MIT Press Reader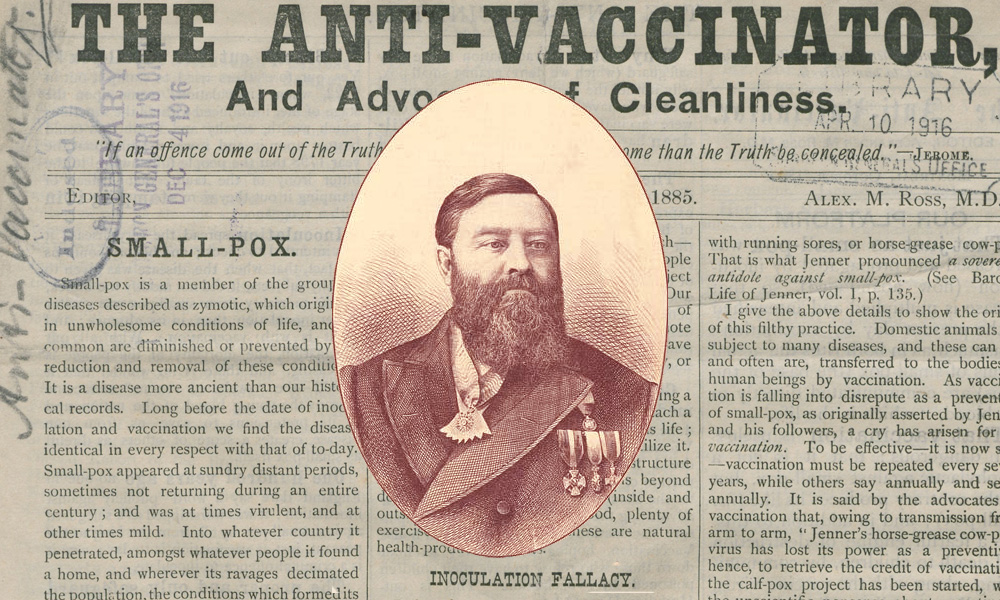
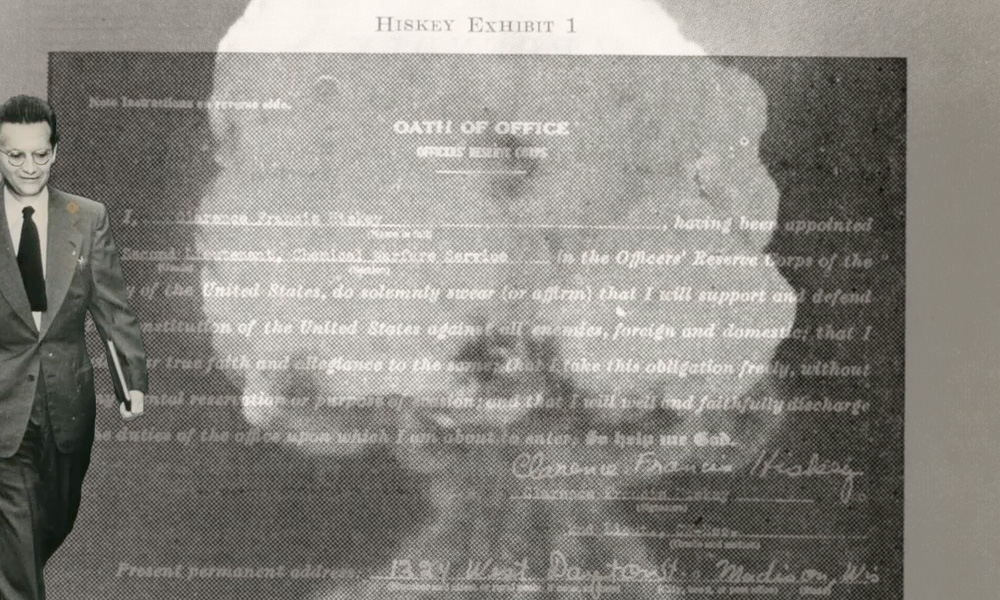
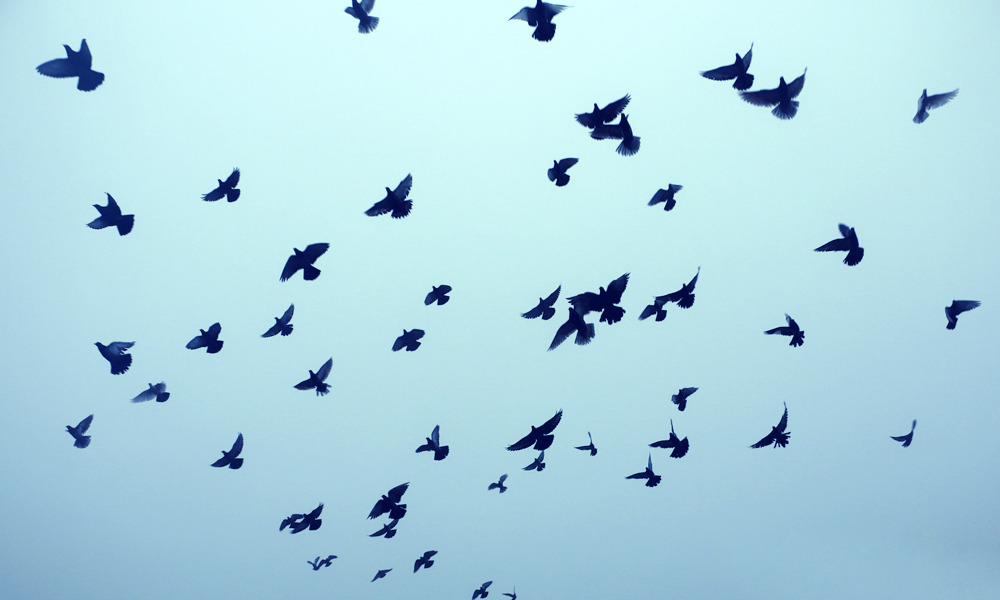
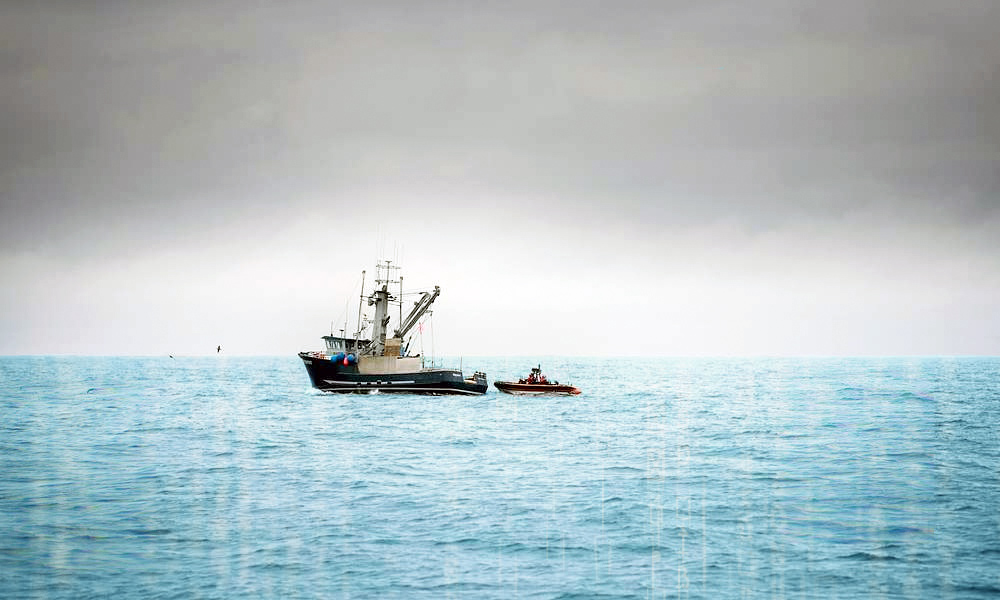
Management on the Cutting Edge

MIT Sloan Management Review and the MIT Press have joined forces to explore the digital frontiers of management. Books in the “Management on the Cutting Edge” series present original research from leading lights in academia and industry, providing practical advice to business leaders on how to prepare for the exciting — and challenging — future that awaits us. Series editor: Abbie Lundberg
New in the series, From Intention to Impact shows what organizations, leaders, and people at all levels must do to create more inclusive environments that honor and value diversity. Malia C. Lazu shares a seven-stage guide through this process as well as a 3L model of listening, learning, and loving that readers can use from the initial excitement of doing “something” to the frustration when the inevitable pushback comes, and finally to the determination to do the hard work despite the challenges—on corporate and political fronts.
Latest News
Read our blog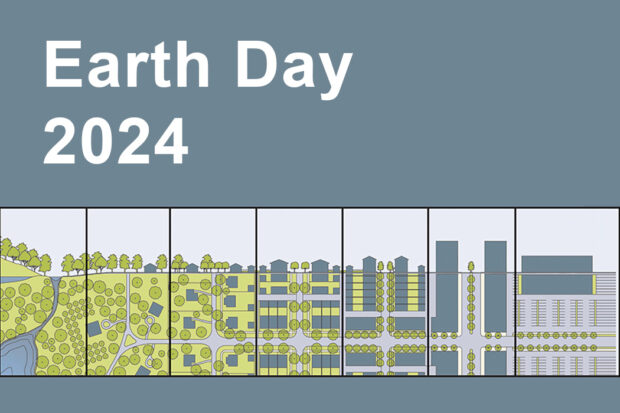
Earth Day 2024: Building a sustainable future
Spotlighting environmental justice and urban design for Earth Day.
Monday April 22, 2024